Digital data management
Digital transformation is increasingly entering classical engineering disciplines. Especially in materials science and engineering, groundbreaking innovations due to modern methods of data processing emerge. That is why the research group Digital Engineering addresses concepts to sustainably digitalize the management of data originating from the various research areas at EMI.
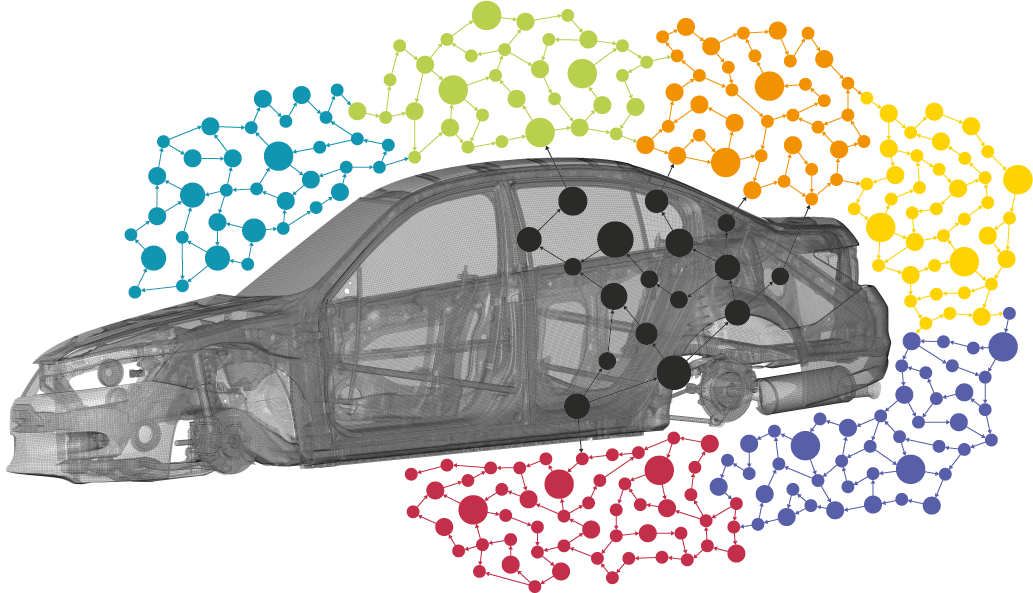
FAIR data: findable – accessible – interoperable – reusable data
In recent years, the pace how heterogeneous and unstructured data are generated worldwide has dramatically accelerated. In addition to their inaccessibility, the bigger part of data assets is not reusable because they are poorly annotated and lack rich metadata. Especially in the light of increasingly data-driven research approaches, the large quantity of information contained in data assets is of crucial value to future R&D activities. The greater goal of the scheme is therefore to address the entire life cycle of research data and exploit it by the consistent implementation of the FAIR guiding principles. To this aim, metadata play a superior role as they form the base to describe and exploit the meaning of data. Semantic technologies allow for the integration of metadata as context information in order to harness the knowledge from the underlying data.